国家禁止课外补课(禁止校外补课)
在教育领域,国家政策始终占据着重要的位置。近年来,随着对教育质量的高度重视和对减轻学生课业负担的呼声日益高涨,“国家禁止课外补课”这一政策应运而生。该政策的出台不仅反映了政府对于当前教育现状的深刻反思,更体现了对未来教育发展的长远考量。本文将围绕“国家禁止课外补课”这一主题展开讨论,旨在深入解析该政策的背景、目的、效果及其带来的影响。
我们需要了解“国家禁止课外补课”这一政策的背景。随着社会的不断发展和进步,人们对教育的需求也在不断提高。传统的应试教育模式使得学生的学习负担不断加重,学生的身心健康受到严重影响。为了解决这一问题,政府开始关注到课外补习的弊端,并逐渐认识到需要从根本上改善教育环境。因此,“国家禁止课外补课”这一政策的出台,正是在这样的背景下应运而生。
我们来看一下该政策的具体目的。国家制定“国家禁止课外补课”政策的主要目的是为了减轻学生过重的课业负担,促进学生全面发展,提高教育质量。同时,通过减少课外补习,可以缓解家长对子女教育的焦虑情绪,营造更加和谐的教育环境。此外,这一政策还有助于推动教育公平,为所有学生提供均等的学习机会。
那么,“国家禁止课外补课”政策的效果如何呢?经过一段时间的实施,我们可以看到,这一政策的实施取得了一定的成效。学生的学习负担明显减轻,学生有更多的时间用于休息、娱乐和参与其他有益的活动。学生的心理健康得到了一定程度的改善。教育质量也有所提高,教师更加注重教学方法的创新和学生的个性化发展。当然,我们也应看到,这一政策的实施并非一帆风顺,仍存在一些问题和挑战,例如如何确保政策的有效执行、如何平衡教育资源的合理分配等。
我们来看看“国家禁止课外补课”政策所带来的影响。这一政策的实施使得教育环境变得更加公平。以往,一些家庭因为经济条件或其他原因无法为子女提供足够的学习资源,而现在,国家的政策为他们提供了平等的机会。这一政策的实施有助于培养学生的自主学习能力和创新能力。学生不再依赖于课外补习来提高成绩,而是更多地依赖于自己的努力和实践。这一政策的实施有助于促进家庭教育的发展。家长不再过度依赖课外补习,而是更加注重与孩子的沟通和陪伴。这对于培养孩子的综合素质和能力具有重要意义。
“国家禁止课外补课”政策是针对当前教育现状而制定的有力措施。它旨在减轻学生过重的课业负担、促进学生全面发展、提高教育质量以及推动教育公平。虽然在实施过程中遇到了一些问题和挑战,但我们应该看到其积极的一面。未来,我们期待政府能够不断完善相关政策,为学生提供更好的教育环境和机会。同时,家长和教师也应该积极响应政策要求,共同为培养德智体美劳全面发展的社会主义建设者和接班人而努力。
1.1 Introduction In the realm of artificial intelligence, there is a growing interest in exploring the application of deep learning algorithms on various fields such as computer vision, natural language processing, and image processing. One area where deep learning has made significant progress and has been widely applied is in the field of speech recognition. This technology has enabled us to convert human speech into digital text, which can then be used for various applications such as automated voice assistants. Speech recognition is an important tool that enables us to communicate with machines in real-time. It allows people to use their voices as input for devices such as smartphones, computers, or smart speakers. The ability to recognize spoken words and sentences is essential for many applications, from customer support chatbots to virtual personal assistants. The development of deep learning models for speech recognition has been rapid in recent years. These models have achieved state-of-the-art performance in terms of accuracy, speed, and efficiency in recognizing speech signals. Deep learning models are capable of capturing intricate patterns and complex relationships within speech signals, allowing them to learn more nuanced representations of spoken language. However, despite the advancements made in deep learning for speech recognition, there are still limitations and challenges that need to be addressed. One of these challenges is noise interference, which can drastically reduce the quality of the output when the model is exposed to noisy speech. Noise can come in various forms, including background noise, environmental factors, and even human error during recording. Noise can significantly affect the accuracy of the speech recognition system by introducing errors in the audio signal. This is because the neural network relies on accurate and consistent inputs to make predictions. If the input data is corrupted due to noise, the model may fail to make correct predictions, leading to poor recognition results. Furthermore, training a deep learning model on speech data requires a large amount of data. To achieve high performance, it is necessary to collect and preprocess a large volume of speech samples. However, collecting such data can be time-consuming and resource-intensive, making it challenging for researchers and developers to work with. Another challenge faced by deep learning models for speech recognition is the complexity of the speech signal. Speech signals consist of multiple layers of information, ranging from phonemes, syllables, to entire words and phrases. Each layer represents a different level of complexity in the language being understood. Despite these challenges, research in deep learning for speech recognition continues to grow rapidly. With ongoing efforts in algorithm optimization, hardware acceleration, and model architecture improvements, we are expected to see further advances in the future. Additionally, advancements in machine learning and deep learning techniques will likely lead to more efficient solutions for speech recognition systems. Overall, the future of deep learning for speech recognition remains bright and promising for continued development and application.本文系作者个人观点,不代表本站立场,转载请注明出处!
戴氏教育精品课程
-
热门高考全科冲刺集训班
适合对象:应届高三期望进入理想院校的学生
课程简介:针对高考毕业生短期全科冲刺
在线咨询 -
特训艺考文化课集训班
适合对象:高三艺体期望进入理想院校的学生
课程简介:针对艺考生短期全科文化冲刺
在线咨询 -
热门中考集训班
适合对象:应届初三期望进入理想院校的学生
课程简介:针对初三毕业生短期全科冲刺
在线咨询 热门高考复读全科冲刺班
适合对象:应届、往届高三/高考参加复读生
课程简介:针对高考复读生短期全科冲刺
在线咨询-
热门高职单招培训班
适合对象:职高生/普高全科学生
课程简介:文化课+面试一体化培训课
在线咨询 -
特训小学补习班
适合对象:小学1-6年级全科孩子
课程简介:针对小学语数外补习课程
在线咨询 -
热门初中辅导班
适合对象:初中1-3年级全科孩子
课程简介:针对初中孩子全科补习课程
在线咨询 热门高中辅导补课班
适合对象:高中1-3年级全科学生
课程简介:针对高中全科辅导集训课程
在线咨询
戴氏中高考学校教学优势
私人订制学习体验
6步助力目标达成
-
诊断问题
先诊断后上课,
找到学科症结是关键 -
规划目标
结合测评结果,
分数差距制定阶 -
匹配师资
根据学员情况,
匹配筛选出更适合孩子的老师 -
跟踪辅导
课前教案调整中,
查漏补缺课后陪读答疑 -
定制方案
根据提升目标,
学科弱项制定专属学习方案 -
实时反馈
阶段性学习反馈,
老师定期和家长沟通学习状况
戴氏冲刺班型设置
戴氏精品课程中心
戴氏DSE教学法 助你圆梦理想学校
温馨提示 | 班型 | 人数 | 课程特色 |
根据学生各科目学习情况, | VIP1对1 | 1人 | 量身定制辅导方案 |
在小班或中班就读期间, | 精品班 | 2-6人 | 因材施教,分层教学 |
针对较弱科目采用一对一辅导形式, | 大师班 | 10余人 | 关注每个学生,夯实基础 |
有针对性的夯实基础、查漏补缺,单科成绩稳步提高。 | 免费学情诊断,定制学习方案 |
戴氏教育西南直营教学点
戴氏教育直营校区
点击下面直营教学点了解详细地址/收费/师资配备
戴氏教育专注中高考培训
戴氏教育郑重承诺
20多年专注中高考精品小班、1对1教学

学习不满意
交多少退多少

入学签订
辅导协议

教学不满意
随时换老师
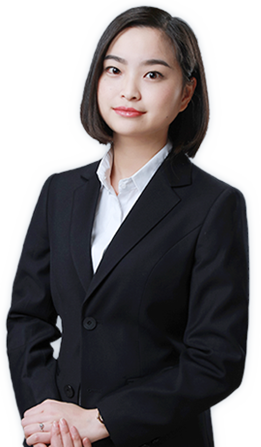